MLOps Services
Where AI Thrives, Innovate With Advanced MLOpsbn USD
The global MLOps market is projected to reach USD 10.8 billion by 2025%
87% of organizations are planning to use MLOps in the next 12 months%
MLOps can reduce the time to deploy ML models by up to 70%%
MLOps can reduce the cost of ML development and operations by up to 50%Streamline Your Machine Learning Operations With Our MLOps Expertise
Experience a paradigm shift in how you approach machine learning projects through our transformative MLOps as a service. Our team of dedicated MLOps specialists aims to propel your AI initiatives to unparalleled heights. Building upon the proven principles of DevOps, we seamlessly infuse machine learning into your operational fabric, ensuring a frictionless journey from development to deployment.
Imagine a landscape where the journey from concept to reality is efficient and showcases streamlined precision. With our MLOps services, this vision becomes a reality. Guided by the DevOps philosophy of collaboration, automation, and iterative enhancement, we align your machine-learning endeavors with operational excellence. This synergy ensures a swift, automated, and dependable transition of your models into real-world solutions. The result is a future where machine learning isn’t just a tool but a dynamic force that propels your organization’s growth.

How Do We Help?
Elevate your machine learning initiatives with our specialized MLOps solutions, overcoming the MLOps challenges that can arise in AI deployments. We craft a tailored strategy to seamlessly integrate machine learning into your operations, optimizing efficiency and effectiveness. With automated workflows, continuous integration and deployment excellence, real-time monitoring, scalability solutions, and robust security measures, we ensure your AI initiatives thrive in a future-ready environment. Partner with us to unlock a new era of AI possibilities and drive exceptional business growth.
MLOps Services
Manage Your Machine Learning Operations with Veritis MLOps Services
End-to-end MLOps Deployment
Seamlessly transition your AI models from development to real-world application. We guide you through data preparation, model training, and deployment, ensuring consistent and reliable performance.
Automated Workflows for AI
Embrace the power of automation with our cutting-edge workflow orchestration. Our custom-built pipelines automate model training, testing, and deployment, reducing manual intervention and minimizing errors.
Continuous AI Evolution (CAIE)
Stay at the forefront of AI advancements with our Continuous Integration and Continuous Deployment strategies. These keep you agile, allowing swift and secure updates and enhancements to your AI models.
Real-time AI Vigilance
Our monitoring solutions vigilantly watch over your AI models in real-time, tracking critical metrics and triggering alerts for anomalies. This ensures the prompt detection and resolution of issues.
AI Model Version Control
Harness the power of version control for your AI models. Effortlessly manage different versions, compare results, and revert as needed, empowering collaboration and controlled experimentation.
Optimized AI Infrastructure
Navigate the complexities of AI infrastructure with our expertise. We tailor cloud and on-premises setups to match your AI model requirements, ensuring scalability and cost-effectiveness.
MLOps Workflow Management
Streamlining Model Lifecycle With Seamless MLOps Workflow Management
Create Advanced ML Workflows and Models
Create intricate ML workflows and cutting-edge models using datasets and model registries. Enhance traceability with comprehensive run history, including code, data, and metrics. Develop elaborate pipelines to craft, deploy, and manage reproducible model workflows for consistency.
Rapidly Deploy Highly Accurate Models
Efficiently utilize managed online endpoints for rapid deployment on powerful CPU and GPU machines. Employ swift packaging, upheld by model profiling and validation MLOps tools, ensuring quality. Facilitate controlled rollouts for seamless model transitions to production environments.
Streamline the Entire MLOps Lifecycle
Efficiently oversee the entire MLOps lifecycle with integrated tools like GitHub Actions. Optimize training, deployment, and CI/CD adherence. Enhance models iteratively with advanced data-drift analysis.
Implement Robust Governance Across Assets
Implement robust governance for model assets. Maintain detailed version history and lineage for audits. Enforce quotas and rules for security, privacy, and compliance. Utilize advanced features for transparency, control, and fairness.
Harness Interoperability for Flexible Workflows
Utilize MLflow for flexible, secure ML workflows across the cloud and edge. Centralize experiments, metrics, and artifacts for a unified workspace. Extend from local to global execution paradigms seamlessly.
Advance Collaborative MLOps Across Workspaces
Rapidly boost cross-workspace collaborative MLOps using registries. Centralize assets for broad access and enable seamless cross-workspace model sharing, deployment, and traceability. Streamline diverse team efforts while ensuring comprehensive transparency.
Comparison Between MLOps and DevOps
MLOps and DevOps share some common principles, like automation and collaboration, but they differ in their focus and the specific challenges they address in their respective domains. MLOps is tailored to the unique requirements of machine learning models, while DevOps is designed to optimize software development and delivery processes.
Aspect | MLOps | DevOps |
Focus | Machine Learning (ML) operations and models | Software development and IT operations |
Purpose | Streamline ML workflows, deployment, and ops | Optimize software development, deployment, and ops |
Main Components | Data pipelines, model registries, monitoring | Code repositories, CI/CD pipelines, Infrastructure |
Data Handling | Deals with ML-specific data and models | Manages code and application-related data |
Core Objective | Improve ML model deployment and management | Accelerate software delivery and reliability |
Key Tools | Kubeflow, MLflow, TensorFlow Extended (TFX) | Jenkins, GitLab, Travis CI, Docker, Kubernetes |
Pros of MLOps
Optimized ML Workflows
MLOps streamlines the end-to-end machine learning process, enhancing the efficiency of data preprocessing, model training, and deployment.
Improved Model Management
MLOps provides robust tools and practices for version control, model tracking, and governance, ensuring better model management.
Real-time Monitoring
MLOps enables continuous model monitoring, quickly detecting performance issues and drift in production.
Scalability
MLOps solutions often include scalability features for handling large datasets and complex models.
Domain-specific Tools
MLOps offers specialized tools like Kubeflow and ML flow tailored to machine learning needs, enhancing productivity.
Optimized ML Workflows
MLOps streamlines the end-to-end machine learning process, enhancing the efficiency of data preprocessing, model training, and deployment.
Improved Model Management
MLOps provides robust tools and practices for version control, model tracking, and governance, ensuring better model management.
Real-time Monitoring
MLOps enables continuous model monitoring, quickly detecting performance issues and drift in production.
Scalability
MLOps solutions often include scalability features for handling large datasets and complex models.
Domain-specific Tools
MLOps offers specialized tools like Kubeflow and ML flow tailored to machine learning needs, enhancing productivity.
Cons of MLOps
Complexity
Implementing MLOps can be complex, especially for organizations new to machine learning, requiring a learning curve.
Data Challenges
Managing ML-specific data and ensuring data quality can be challenging and time-consuming.
Resource Intensive
Setting up and maintaining MLOps infrastructure may require significant resources, both in terms of hardware and skilled personnel.
Model Version Control
Keeping track of different versions of machine learning models and ensuring consistency across environments can be challenging, potentially leading to version control issues.
Continuous Monitoring Overhead
Real-time model monitoring can strain computing resources and increase operational costs, especially in high-throughput scenarios, affecting scalability.
Roadmap for a Successful MLOps
Navigating the Future - Your Guided Roadmap to Achieve MLOps Excellence
- Leadership commitment is crucial for aligning the organization with MLOps practices.
- Identifying resource needs and creating a strategy sets the foundation for a successful implementation.
- Creating a streamlined workflow that integrates development, deployment, and monitoring ensures a cohesive approach.
- Implementing version control guarantees model traceability and reproducibility, critical for accountability and compliance.
- Automated deployment pipelines improve deployment speed and consistency.
- Automation in monitoring and scaling optimizes performance and resource allocation based on real-time metrics.
- Model governance ensures that models are used ethically and within regulatory boundaries.
- Continuous monitoring, auditing, and updates maintain model accuracy and fairness over time.
- Collaboration among cross-functional teams improves communication and understanding between data scientists, developers, and operations.
- MLOps Platforms like Microsoft Teams and Slack facilitate the seamless sharing of insights and knowledge.
- Application performance monitoring MLOps tools identify performance bottlenecks and inefficiencies.
- Optimizing resource utilization leads to enhanced user experiences and efficient operations.
Success Stories
Veritis has empowered numerous clients to achieve exceptional outcomes by implementing MLOps best practices, driving collaboration, efficiency, and accelerated model deployment.
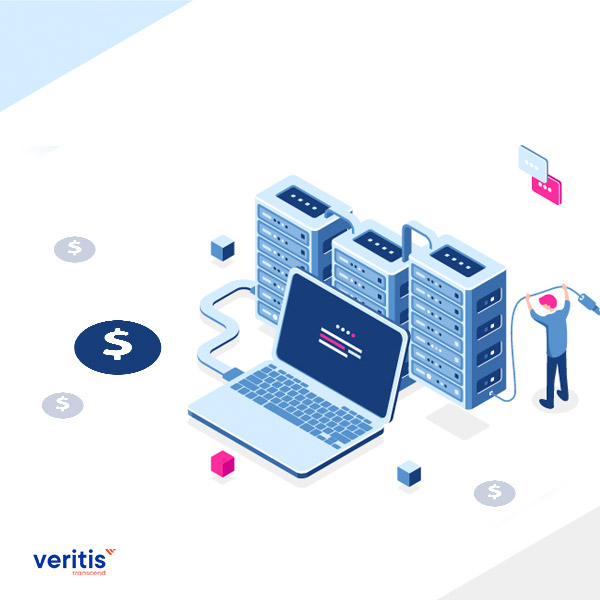
Data Center Migration, Server Builds and IT Infrastructure Support for Banking Industry
Business Value Delivered Association with us helped the client improve their server infrastructure involving both physical...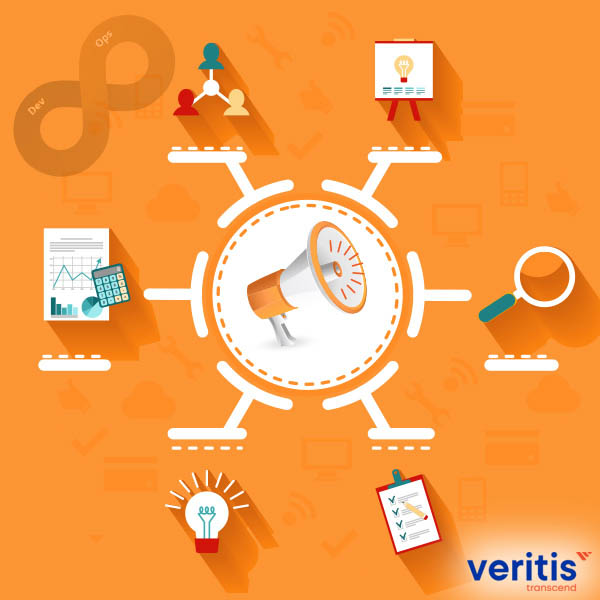
Marketing Solutions Provider Adopts ‘DevOps’
Client Profile One of the fastest growing marketing solutions company headquartered in the United States. The company...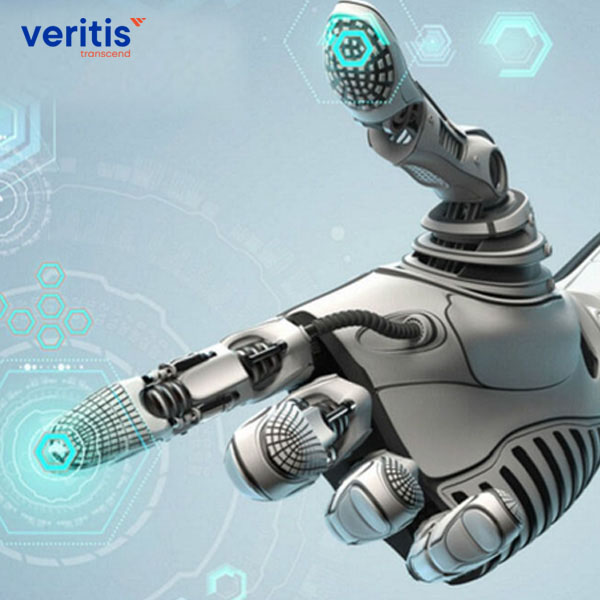
Full-Fledged Infrastructure Automation and Cloud Support to Finance and Insurance Company
Client Information Our client specializes in dealing with the challenges of the insurance, investment and claims industry....Resources
Veritis provides valuable resources and expertise to clients seeking MLOps services, equipping them with streamlined machine-learning operations and industry-leading insights.

MLOps Best Practices: Building a Robust Machine Learning Pipeline
MLOps is more than just a trendy term in the AI and ML community. It has...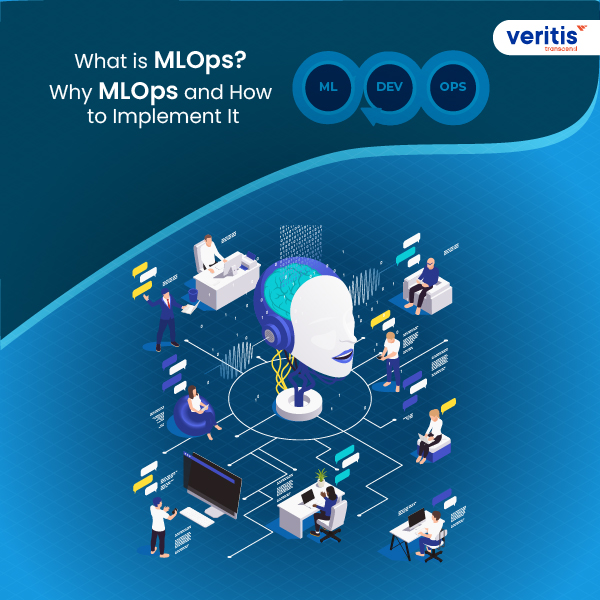
What is MLOps? Why MLOps and How to Implement It
Until recently, we were all engaged in understanding the software development lifecycle (SDLC) and its various...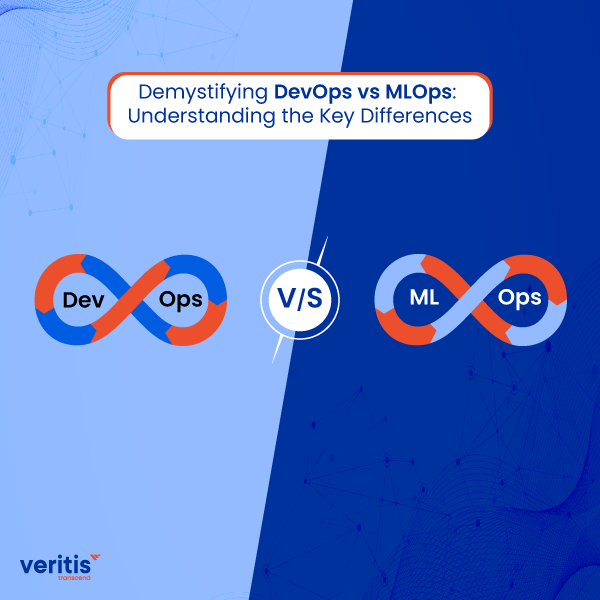